I have been downloading the daily average global surface air temperature data initial condition output for the Sigma 0.995 level from the National Centers for Environmental Prediction and National Center for Atmospheric Research Reanalysis 1 (NCAR R1) cooperative effort. This output is still being updated daily about 2 days behind the current day and extends back to 1948. So far I have downloaded and processed the daily temperature output back to 1979. The Sigma 0.995 level corresponds to the pressure altitude at 99.5 % of the surface air pressure, which is roughly about 50 meters above ground level. The actual height above ground level varies somewhat depending on atmospheric conditions. The NCAR R1 model uses a 2.5 degree latitude by 2.5 degree longitude grid. In this post I am comparing the resulting global mean surface air temperature anomalies (GMSATA) for several time periods and different reference baseline periods. See the Methods section at the end for calculation details and links to sources.
The first two graphs, Figures 1 and 2, display NCAR R1 daily average GMSATA time series output for two different reference baselines. I was expecting the older 1979-2000 baseline to show higher temperature anomalies than the much more recent 2011-2015 baseline as can be seen in the graphs, but I was surprised that the baseline shift also changed the seasonal pattern across each year. Apparently the seasonal patterns have shifted from one baseline period to another. Figure 3 is provided to compare the daily average 2-meter above ground level surface air temperature anomalies from the more modern Climate Forecast System Reanalysis (CFSR) to the NCAR R1 output. The CFSR has a higher resolution of 0.5 degree latitude by 0.5 degree longitude. See the Daily Updates page, which can be accessed from the menu bar at the top of this page, for more information about the daily CFSR output. Click on any of the graphs to see a larger copy.

Figure 1. NCAR R1 GMSATA daily 2018 through 2019 February 17, referenced to 2011-2015 baseline

Figure 2. NCAR R1 GMSATA daily 2018 through 2019 February 17, referenced to 1979-2000 baseline

Figure 3. CFSR GMSATA daily 2018 through 2019 February 18, referenced to 1979-2000 baseline
I also calculated GMSATA for two other reference baseline periods, 1994-2013 and 1981-2010. The time series results for all four reference baseline periods for 2018-2019 are plotted together in Figure 4. They all converge around January-February and diverge the most around September-October. I’m not sure why.
I included the 1994-2013 reference period used by Nick Stokes for reporting daily averages here and the 1979-2000 period used by the University of Maine Climate Reanalyzer here. The 1981-2010 period is the most recent three decade (30-year) period commonly used for climatological data reporting. I previously used the 2011-2015 period for comparing monthly NCAR R1 versus CFSR temperature anomalies here.

Figure 4. NCAR R1 GMSATA 2018 through 2019 February 17 reference baseline comparisons
The next three graphs, Figures 5 through 7, are like the first three graphs, but covering a longer time period, from 2014 to 2019 so far. Again the general patterns are similar, but the details differ.

Figure 5. NCAR R1 GMSATA daily 2014 through 2019 February 17, referenced to 2011-2015 baseline

Figure 6. NCAR R1 GMSATA daily 2014 through 2019 February 17, referenced to 1979-2000 baseline

Figure 7. CFSR GMSATA daily 2014 through 2019 February 18, referenced to 1979-2000 baseline
The last two graphs in Figures 8 and 9 cover a longer time period, for the current century so far, beginning in 2001. I do not yet have CFSR daily averages for all of this period, so only the NCAR R1 results for two different reference periods are presented.

Figure 8. NCAR R1 GMSATA daily 2001 through 2019 February 17, referenced to 2011-2015 baseline

Figure 9. NCAR R1 GMSATA daily 2001 through 2019 February 17, referenced to 1979-2000 baseline
Overall, this exercise goes to show that changing reference baseline periods for daily GMSATA does cause quite a bit of variation in the results – more than I expected. However, the general trends as indicated by the running 365-day averages did not appear to be affected by changing baselines.
Methods
For the NCAR R1 daily averages I downloaded the gridded Sigma 0.995 level temperature output which is provided in compacted annual files (thanks to Nick Stokes for providing the link below on his blog). I used the National Aeronautic and Space Administration (NASA) Panopoly program to extract the temperature grids from the compacted data files and then loaded the daily temperature grid data into spreadsheets by year. For each day I calculated daily averages by latitude zone, weighted by area, to compile zonal and global averages.
I calculated the reference period baseline averages for each day and used centered running 5-day averages to smooth the baseline results. Once the reference baseline temperatures were calculated, the temperature anomalies were calculated for each day by subtracting the reference baseline value for the day from the actual daily temperature average for that day. For the temperature anomalies I also calculated running 365-day and 91-day averages to show annual and seasonal scale tendencies.
NCAR R1 annual compacted files with daily global temperature grids:
ftp://ftp.cdc.noaa.gov/Datasets/ncep.reanalysis.dailyavgs/surface/
NASA Panoply program:
https://www.giss.nasa.gov/tools/panoply/
Update 2019 February 19
I have updated all of the graphs in Figures 1-9 to the latest available date in order to match additional graphs provided below in Figures 10-13. I am adding Figures 10-12 to present graphs of the 1994-2013 baseline results for comparison with corresponding graphs above using 2011-2015 and 1979-2000 baselines.

Figure 10. NCAR R1 GMSATA daily 2018 through 2019 February 17, referenced to 1994-2013 baseline

Figure 11. NCAR R1 GMSATA daily 2014 through 2019 February 17, referenced to 1994-2013 baseline
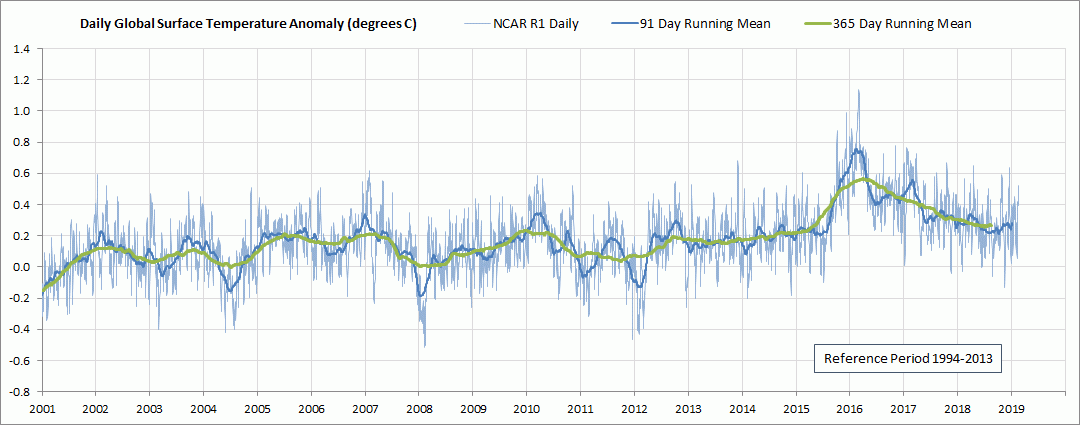
Figure 12. NCAR R1 GMSATA daily 2001 through 2019 February 17, referenced to 1994-2013 baseline
Figure 13 shows the actual baseline global mean surface air temperatures (GMSAT) for consideration regarding Figure 4. As in that figure, the different baselines show a convergence for December through February and a divergence that peaks around August to October. I am not sure how much is a real atmospheric change over time, or how much could be some kind of artificial change induced by variations in the input data over time.

Figure 13. NCAR R1 GMSAT reference baseline comparisons
Figure 14 shows the difference in the GMSAT from the 2011-2015 baseline minus the 1979-2000 baseline. The largest increases since 1979-2000 were from August to October and the smallest increases were in January and February. I am not certain if the distribution of these changes is dominated by real atmospheric changes or if there are significant artificial changes induced by changing data inputs over time that influence the seasonal distribution and magnitudes.

Figure 14. NCAR R1 GMSAT reference baseline difference 2011-2015 minus 1979-2000
As I process additional NCAR R1 output back to 1948, I plan to evaluate and compare some additional reference baselines, including overlapping 3-decade baselines beginning with 1951-1980. I also plan to look at the baseline variations in the hemispheric as well as 30 degree latitude zones and the results will be presented in future posts.
Update 2019 March 1
I finished loading and processing all of the NCAR R1 daily GMSAT data back to 1948 and I am adding below some additional baseline comparisons. Figure 15 compares the effects of using different baselines to evaluate the last year of daily GMSATA, similar to Figure 4 above. Here the baselines compared are overlapping 3-decade periods from 1981-2010 back to 1951-1980.

Figure 15. NCAR R1 GMSATA 2018 through 2019 February 28 reference baseline comparisons back to 1951-1980
Figure 16 compares the actual baseline daily GMSAT averages for all of the baselines I have analyzed so far, including those previously provided in Figure 13 above.

Figure 16. NCAR R1 GMSAT reference baseline comparisons back to 1951-1980
Figures 17 and 18 show the daily GMSAT changes from subtracting different baselines for the periods indicated in each graph.

Figure 17. NCAR R1 GMSAT reference baseline difference 2011-2015 minus 1951-1980
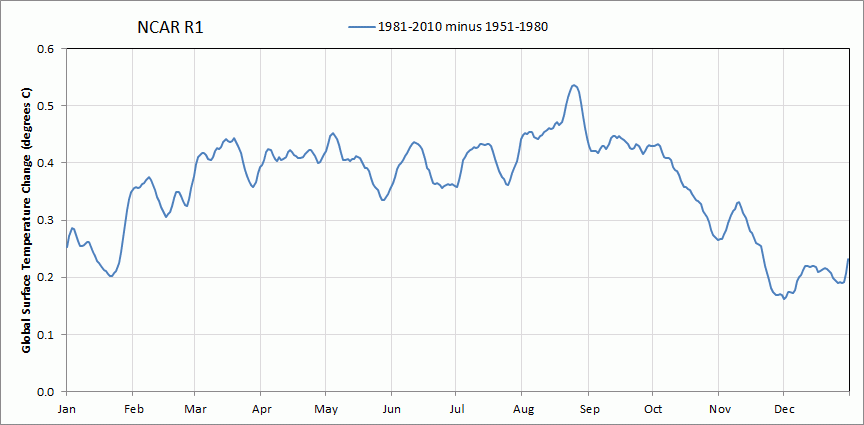
Figure 18. NCAR R1 GMSAT reference baseline difference 1981-2010 minus 1951-1980
In conclusion, it is apparent that changing baselines does effect the seasonal patterns in the daily GMSATA results, but does not effect the general annual patterns as indicated by the running 365-day averages.
Update 2019 March 2
I’m adding two animated GIF graphs to better illustrate my conclusion above. The animations in Figures 19 and 20 compare NCAR R1 daily GMSATA referenced to 2011-2015 in comparison to 1994-2013 and 1951-1980 baselines respectively. Notice that the running 365-day average pattern does not change as the baseline changes, but the seasonal patterns change significantly. I suspect that some of the seasonal changes are real and at least part of the changes are artificial because of changing data inputs over time (especially for periods prior to satellite observations, such as the 1951-1980 baseline). Click on either figure to see a larger copy.

Figure 19. Animation comparing the NCAR R1 GMSATA for 2001-2019 using two different reference baseline periods, 2011-2015 versus 1994-2013

Figure 20. Animation comparing the NCAR R1 GMSATA for 2001-2019 using two different reference baseline periods, 2011-2015 versus 1951-1980
Update 2019 May 5
Here are two more graphs comparing GMSAT changes from one baseline to another. Notice that the largest increases in GMSAT have been in the August to October range, with the lowest increases in December and January. Click on either figure to see a larger copy.
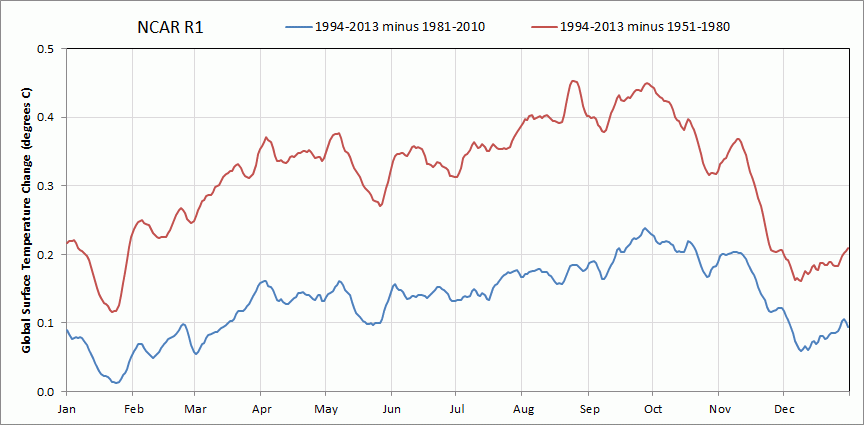
Figure 21. NCAR R1 GMSAT reference baseline difference 1994-2013 minus 1981-2010 and 1994-2013 minus 1951-1980

Figure 22. NCAR R1 GMSAT reference baseline difference 1981-2010 minus 1951-1980